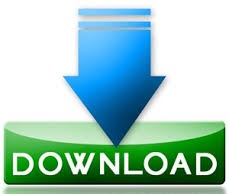

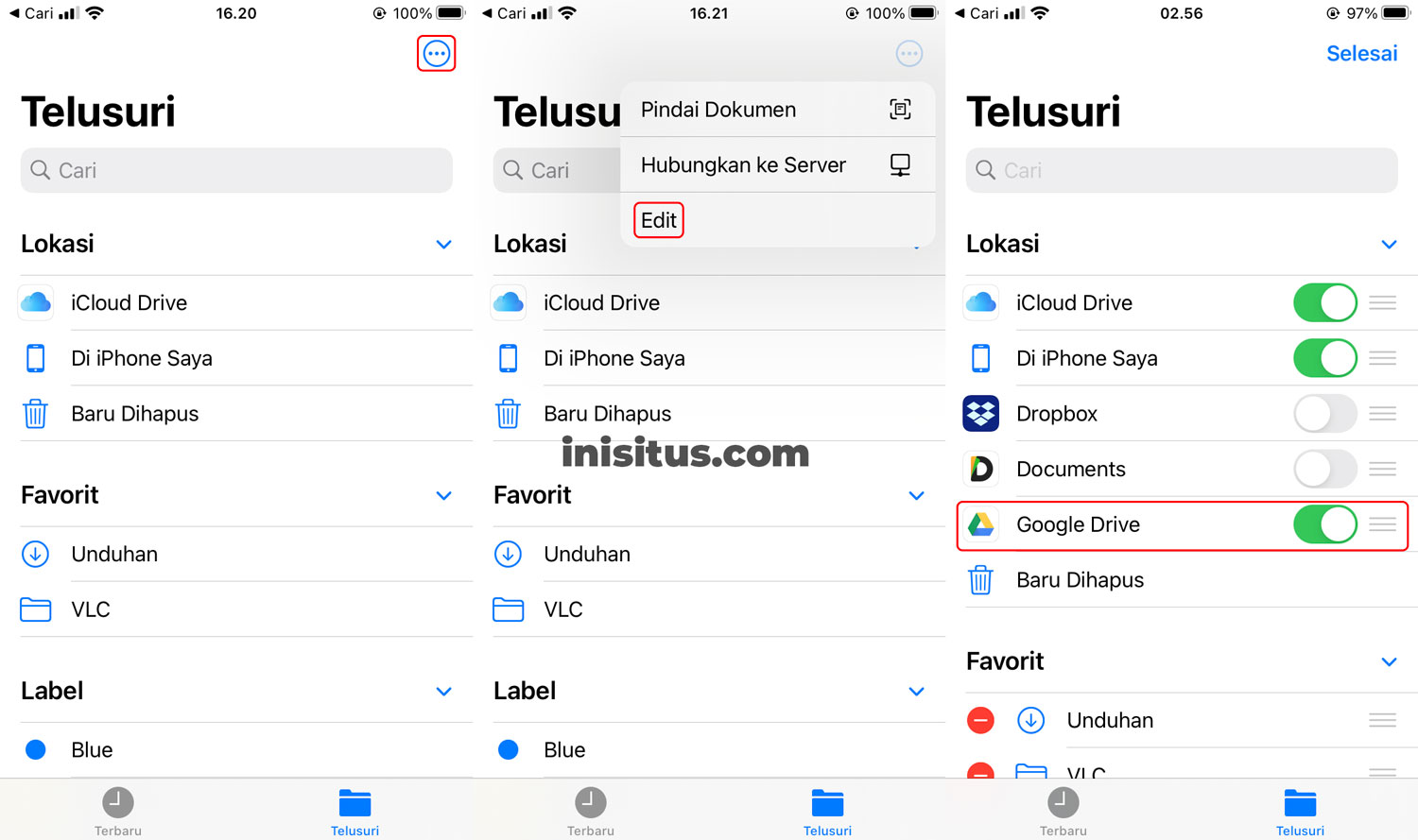
Regardless of what happens to your device, your files are stored safely in Google Drive.
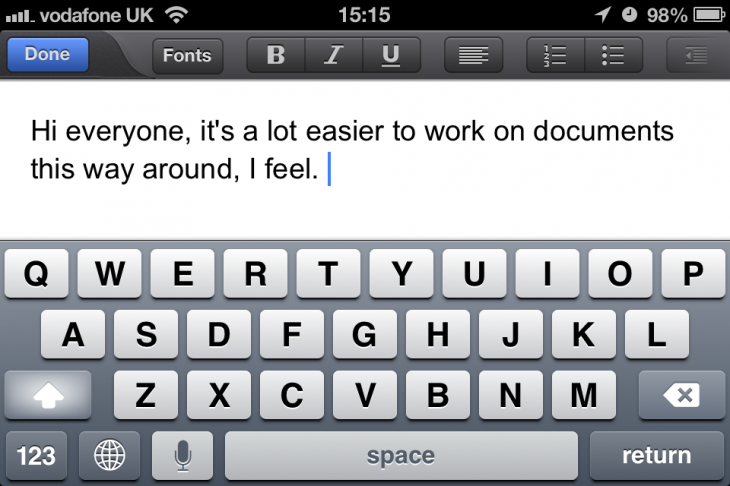
In addition, Google Drive also supports users view more than 30 types of files right in your browser including HD video, Adobe Illustrator and Photoshop even if you do not install the program on your computer.įor those who work in office, Google Documents, Pages and Presentation Pages are available in Drive.
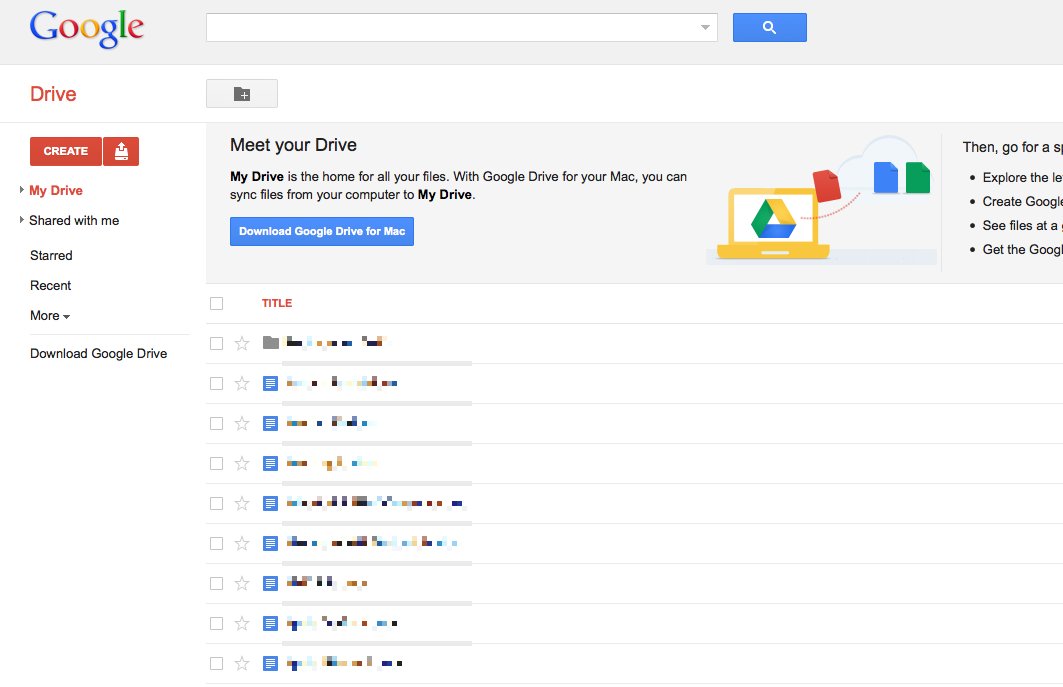
Google Drive helps you access your file faster by recognizing the objects in your image and text in the scanned documents.Įasy to download your content in Google Drive, just drag one or hundreds of files into the Drive folder on your computer and they will always be available at. Download photos, videos, documents, and other files that are important to you up to Google Drive. Even if your computer, phone or tablet is broken, the files you store in the Google Drive are safe. Our work closes the gap between the theory and the practice in artificial intelligence, in a sense that it confirms that it is possible to learn with very small error allowed.Using Google Drive is a great way to store your files safely in secure data centers, where your files will not be lost. This is also possible with invariant networks that are also universal approximators. To make it happens, we propose a novel optimization framework for our Bayesian Shallow Network, called the learning and invariance descriptor tools, to always reach 100\% accuracy. This is also the case of the Shallow Gibbs Network model that we built as a Random Gibbs Network Forest to reach the performance of the Multilayer feedforward Neural Network in a few numbers of parameters, and fewer backpropagation iterations. They can self-classify many high dimensional data in a few numbers of mixture components. There is a great advantage to build a more powerful model using mixture models properties. His hyper-parameters, the learning rate, the batch size, the number of training times (epochs), the size of each layer, the number of hidden layers, all can be chosen experimentally with cross-validation methods. This model can easily be augmented to thousands of possible layers without loss of predictive power, and has the potential to overcome our difficulties simultaneously in building a model that has a good fit on the test data, and don't overfit. The classical Multilayer Feedforward model has been re-considered and a novel $N_k$-architecture is proposed to fit any multivariate regression task. The tools are given through the chapters that contain our developments. We have performed in this thesis many experiments that validate this concept in many ways. We mean a learning model that can be generalized, and moreover, that can always fit perfectly the test data, as well as the training data.
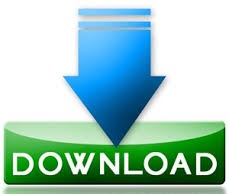